Python primer for HTMD#
Why Python?#
Modern, complete and open-source programming language
Wide spread throughout the scientific community
Easy to understand, rapid delivery and maintenance
C++ still wins on low latency and control of memory
However, Python is versatile and has packages that can be as fast as C (e.g. NumPy)
HTMD relation to Python and Miniconda#
HTMD is written in Python, using the Python 3 synthax
HTMD is, and works as, a Python module.
HTMD is distributed through a package/module distribution platform called Miniconda (light-weight Anaconda)
Advantages of Miniconda:
Already distributes Python (
python
) and interactive Python (ipython
) interpretersEverything is self-contained inside Miniconda and one does not have to rely on the operative system
Programming basics with Python#
Python can do pretty much what any other programming language can. Arithmetics, variable definition, printing, etc.
a = 3 + 2
print('a is', a)
a is 5
Python Interpreters and writing Python code#
python
is the general interpreterOne can write a
script.py
file in Python and runpython script.py
There are powerful ways of writing and prototyping code in Python:
Interactive interpreters (
ipython
,jupyter
)IDE’s (Spyder, Pycharm)
Jupyter Notebooks#
This presentation is actually a Jupyter notebook (you can play with it, run the code yourself)
A notebook like this one is a grapical interactive environment.
It is made of cells:
Text cells, with formatted markdown text (like the bulleted text we have here)
Code cells, to run code interactively
Extra: IPython built-in magic commands#
In IPython, some magic commands are available/built-in.
Useful to navigate the filesystem (% indicates a magic command)
%ls
%mkdir test
Or for module reload for rewritting code for testing without need to reload the module all the time
%load_ext autoreload
%autoreload 2
Python modules usage#
A Python module is a file containing Python definitions and statements.
In Python, modules have to be imported in order to be used.
Here we show two ways of importing modules and one way of importing a functionality:
import os
import numpy as np
from glob import glob
In the first two, using the module funcionalities require the namespace:
the original one os
, or the defined one np
(instead of
numpy
). In the last one, glob
can be used instead of
glob.glob
os
module and glob
function: interacting with the filesystem#
The
os
module serves to interact with the operative systemNormal filesystem commands or magic commands work from the interactive session, but, in a program, the
os
functions should be used instead
os.chdir('.') # change directory
os.listdir('.') # list contents of directory
glob('python-*') # returns pathnames matching a regex pattern
['python-primer.ipynb', 'python-primer.slides.html']
General Programming Overview#
Strings#
Strings are identified by single or double apices:
b='newtest'
c="newtest"
Relational operators#
Relational operators are ==
,<
,>
,<=
,>=
, and
!=
, and they can be connected with and
,or
,not
b == c
True
Lists of objects (integers, strings…)#
Lists of different objects are created using square brackets
d = [1, 2, 3, 'ba', 5, 6, 7, 8, 9]
print(d[3])
ba
Note: in Python, indexes are zero-based.
Conditionals (if-clause)#
if 1 > 0:
d = 100
print(d)
100
As you probably know, in Python, indentation is important. Use
indentation to define a scope of code after the :
. print(d)
is
no longer inside the scope of the if-clause because it is not
indented.
Loops (for-loop)#
for i in [1,2,3,4,5]:
print(i)
1
2
3
4
5
Another way of doing the same, using Numpy:
for i in np.arange(1,6):
print(i)
1
2
3
4
5
Numpy: the best way to handle arrays#
Arrays are best created and handled using numpy
arrays (no need to
import the module, we already did before)
m = np.array([[ 11., 12, 13, 14 ],
[ 21, 22, 23, 24 ],
[ 31, 32, 33, 34 ],
[ 41, 42, 43, 44 ]
]
)
print(m)
[[ 11. 12. 13. 14.]
[ 21. 22. 23. 24.]
[ 31. 32. 33. 34.]
[ 41. 42. 43. 44.]]
where m
is 4-by-4 matrix of double numbers (and not integers), due
to the fact than 11.
is a real number (notice the dot).
Array multiplication#
Arrays can be multiplied easily element by element
n = 3.0 * m
print(n)
[[ 33. 36. 39. 42.]
[ 63. 66. 69. 72.]
[ 93. 96. 99. 102.]
[ 123. 126. 129. 132.]]
A scalar multiplication is applied to each element of the array.
Sequences#
An array sequence can be created with arange
(like seen before).
Array indexing starts at 0.
o = np.arange(0,4)
print(o)
[0 1 2 3]
Slicing#
o[1:] #from 1 until the end, (starts at zero)
array([1, 2, 3])
Note: See how we got the outcome without printing? It’s because we’re in interactive Python
m[:,0] = 99 # first column
m[-2:,] # backwards indexing is possible, getting the last 2 rows
array([[ 99., 32., 33., 34.],
[ 99., 42., 43., 44.]])
More matrix operations#
np.multiply(m,o)
array([[ 0., 12., 26., 42.],
[ 0., 22., 46., 72.],
[ 0., 32., 66., 102.],
[ 0., 42., 86., 132.]])
m.transpose()
np.inner(m,o)
array([ 80., 140., 200., 260.])
Other numpy operations#
np.concatenate((o,o))
array([0, 1, 2, 3, 0, 1, 2, 3])
m.sum(axis=0)
array([ 396., 108., 112., 116.])
print(o.shape)
print(o.size)
print(o.ndim)
(4,)
4
1
Functions#
Subroutines are defined using def
:
def test(a, b=1, c=3):
return a*b*c
test(1, c=5)
5
Variables and Objects#
Python represents all its data as objects.
Variables are just names.
Some objects are mutable, some immutable.
Immutables are: int, float, complex, str, tuples, bytes, frozensets
Mutables are: list, byte array, sets, dict, classes
Identity of an object#
With id
it is possible to check the unique identity of an object
n = 1 # immutable integer
id(n)
140351964294272
n += 1
id(n) # new object
140351964294304
m = [1] # mutable list
id(m)
140351546176392
m.append(2)
id(m) # same object
140351546176392
Argument passing#
Passing an argument to a function is like creating a new name to the object
If it is mutable, then any change inside the function will affect the object outside.
If it is immutable and the function changes it, then python creates another object inside the function scope, so nothing changes outside of the function.
Plots using matplotlib
module#
from matplotlib.pylab import plot,title,xlabel,ylabel,grid,show,savefig,gcf
#Optional: magic line to make plots inline
%matplotlib inline
x = np.arange(0,50,.5)
y = np.sin(x/3) - np.cos(x/5)
plot(x,y, '.-k')
plot(x,np.sin(x/2) - np.cos(x/5),'.r')
title('A simple double plot')
xlabel('variable 1'), ylabel('variable 2'), grid(True)
fig = gcf() # Get current figure
show()
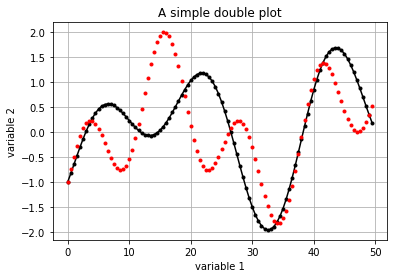
The plot is shown inline, but can also be saved:
fig.savefig('/tmp/foo.eps')
Getting Help#
The help
function provides help for any function:
help(print)
Help on built-in function print in module builtins: print(...) print(value, ..., sep=' ', end='n', file=sys.stdout, flush=False) Prints the values to a stream, or to sys.stdout by default. Optional keyword arguments: file: a file-like object (stream); defaults to the current sys.stdout. sep: string inserted between values, default a space. end: string appended after the last value, default a newline. flush: whether to forcibly flush the stream.
Debugging code in notebooks#
Really nice to debug an error and see what’s wrong by inspecting
variables using %debug
(Press PgDown for example)
def broken_function(b):
print(b,xxx())
c = 4
broken_function(c)
---------------------------------------------------------------------------
NameError Traceback (most recent call last)
<ipython-input-29-441f8be6e164> in <module>()
2 print(b,xxx())
3 c = 4
----> 4 broken_function(c)
<ipython-input-29-441f8be6e164> in broken_function(b)
1 def broken_function(b):
----> 2 print(b,xxx())
3 c = 4
4 broken_function(c)
NameError: name 'xxx' is not defined
%debug
> [0;32m<ipython-input-29-441f8be6e164>[0m(2)[0;36mbroken_function[0;34m()[0m
[0;32m 1 [0;31m[0;32mdef[0m [0mbroken_function[0m[0;34m([0m[0mb[0m[0;34m)[0m[0;34m:[0m[0;34m[0m[0m
[0m[0;32m----> 2 [0;31m [0mprint[0m[0;34m([0m[0mb[0m[0;34m,[0m[0mxxx[0m[0;34m([0m[0;34m)[0m[0;34m)[0m[0;34m[0m[0m
[0m[0;32m 3 [0;31m[0mc[0m [0;34m=[0m [0;36m4[0m[0;34m[0m[0m
[0m[0;32m 4 [0;31m[0mbroken_function[0m[0;34m([0m[0mc[0m[0;34m)[0m[0;34m[0m[0m
[0m
ipdb> quit
Python Exercises#
Sum the first 50 numbers with a for loop
Do the same thing using
numpy
arraysWrite a function that set a value for its argument (that is an integer)
The same but for a
numpy
arrray